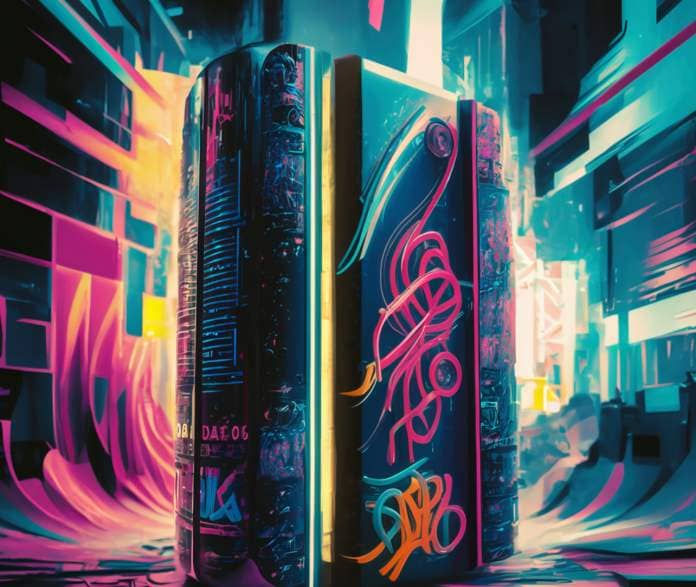
Aspect Sentiment Classification (ASC) is a crucial activity geared toward discerning sentiment polarity inside particular domains, resembling product critiques, the place the sentiment towards explicit elements must be recognized. Continual Learning (CL) poses a big problem for ASC fashions as a consequence of Catastrophic Forgetting (CF), whereby studying new duties results in a detrimental lack of beforehand acquired information. As ASC fashions should adapt to evolving information distributions throughout various domains, stopping CF turns into paramount.
When the variety of duties rises, conventional strategies often require conserving distinct mannequin checkpoints for each activity, which turns into unfeasible. Recent strategies attempt to scale back CF by individually freezing the core mannequin and coaching task-specific elements. However, they often fail to contemplate efficient information switch between duties, which makes it tougher for them to deal with an growing variety of domains successfully.
Recently, a analysis group from China printed a brand new article introducing progressive strategies to deal with the constraints of current approaches in ASC. Their proposed method, Continual Adapter Tuning (CAT), employs task-specific adapters whereas freezing the spine pre-trained mannequin to forestall catastrophic forgetting and allow environment friendly studying of latest duties. Additionally, continuous adapter initialization aids information switch, whereas label-aware contrastive studying enhances sentiment polarity classification. A majority sentiment polarity voting technique simplifies testing by eliminating the necessity for activity IDs, leading to a parameter-efficient framework that improves ASC efficiency.
The proposed CAT technique addresses the problem of sequential studying in ASC duties by leveraging Adapter-BERT structure, a variant of the BERT (Bidirectional Encoder Representations from Transformers) mannequin structure extending BERT by incorporating adapters, that are small neural community modules inserted into every layer of the BERT structure. These adapters enable BERT to be fine-tuned for particular downstream duties whereas retaining most of its pre-trained parameters. Adapter-BERT thus allows extra environment friendly and parameter-efficient fine-tuning for varied pure language processing duties, together with sentiment evaluation, textual content classification, and language understanding duties. In CAT, a separate adapter is realized for every ASC activity, guaranteeing the spine pre-trained mannequin stays frozen to forestall catastrophic forgetting. The course of includes taking enter sentences and side gadgets, producing hidden states, and label-aware options particular to every activity. To improve classification effectivity, a label-aware classifier is developed, integrating contrastive studying to align enter options and classifier parameters in the identical area, leveraging label semantics. Training includes minimizing a mixed loss operate comprising variant cross-entropy and label-aware contrastive loss. Continual adapter initialization methods, together with FinalInit, RandomInit, and ChooseInit, facilitate information switch from earlier duties to new ones. Finally, a majority sentiment polarity voting technique is proposed for testing, eliminating the necessity for activity IDs and offering last sentiment polarity predictions based mostly on voting throughout reasoning paths within the adapter structure. CAT ensures environment friendly and correct sentiment polarity classification in ASC duties via these steps whereas supporting continuous studying and information switch.
The authors evaluated the CAT framework via experiments evaluating it with varied baselines. They used 19 ASC datasets, assessing the accuracy and Macro-F1 metrics. Baselines included each non-continual and continuous studying approaches, with variations for domain-incremental studying. They detailed implementation utilizing BERTbase and adapters. Results confirmed CAT outperformed baselines in accuracy and Macro-F1. Ablation research and parameter effectivity comparisons additional validated CAT’s effectiveness.
In conclusion, the analysis group presents an easy but extremely efficient parameter-efficient framework for continuous side sentiment classification inside a domain-incremental studying context, attaining unprecedented accuracy and Marco-F1 metrics. However, the framework’s applicability past domain-incremental studying settings stays to be explored. This side will probably be addressed in future analysis endeavors.
Check out the Paper. All credit score for this analysis goes to the researchers of this venture. Also, don’t neglect to observe us on Twitter. Join our Telegram Channel, Discord Channel, and LinkedIn Group.
If you want our work, you’ll love our newsletter..
Don’t Forget to hitch our 39k+ ML SubReddit
Mahmoud is a PhD researcher in machine studying. He additionally holds a
bachelor’s diploma in bodily science and a grasp’s diploma in
telecommunications and networking techniques. His present areas of
analysis concern laptop imaginative and prescient, inventory market prediction and deep
studying. He produced a number of scientific articles about person re-
identification and the examine of the robustness and stability of deep
networks.